Development and validation of a Natural Language Processing tool to enable clinical research in emergency and acute care medicine: retrospective cohort study
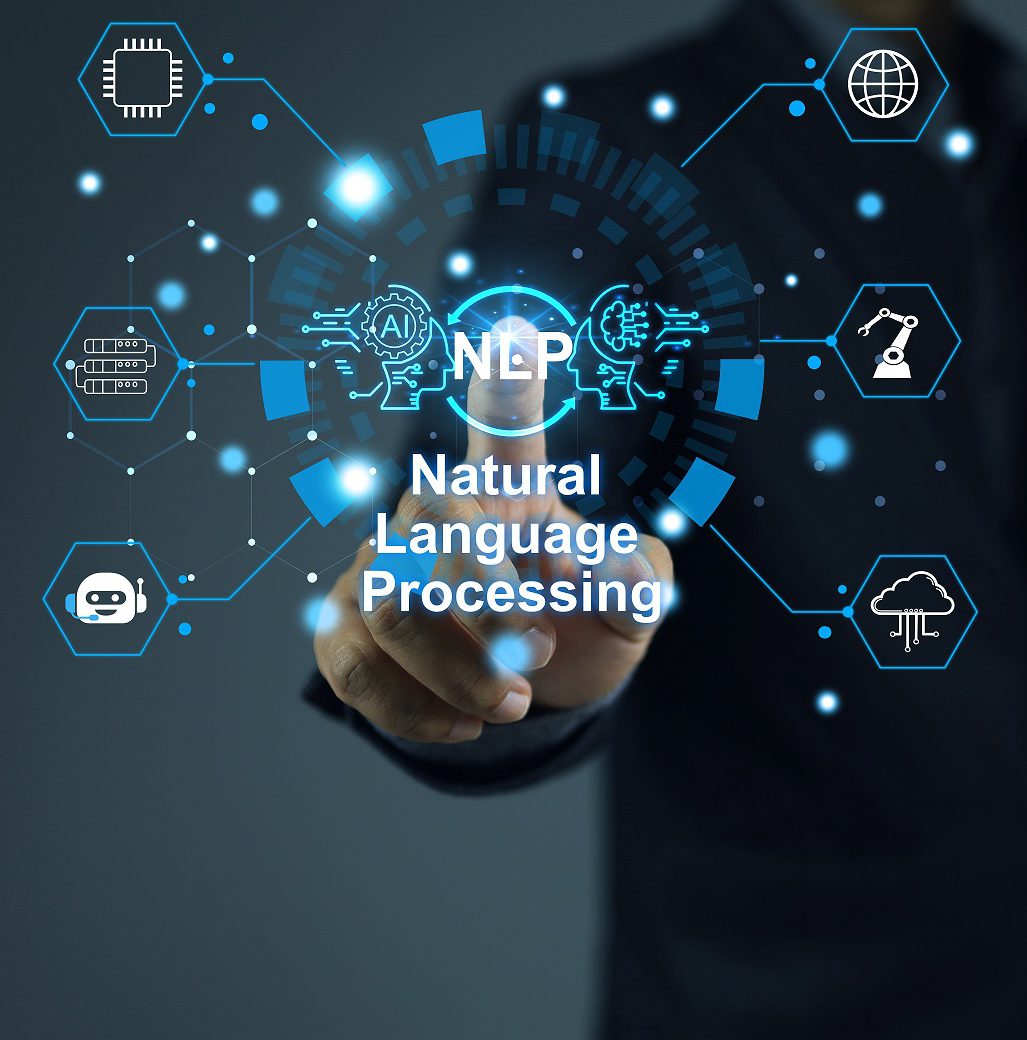
The emergency medicine setting is not favourable to conducting research due to the vast number of patients seen every day and to the chronic staff shortages. The only way to collect data that would permit studying and addressing the areas in need of improvement is to extract such data from emergency department electronic health records (EHRs). This automatic data extraction would avoid dedicated, time-consuming data collection. Obtaining consistent data from EHRs is a complex task, however. While part of the data registered in EHRs is structured (e.g., lab test results and vital parameters) and therefore easy to retrieve, the most useful patient information is often in free text form (e.g., presence of signs and symptoms, suspected and confirmed diagnosis, anamnesis). Such circumstances and needs require a reliable natural language processing (NLP) tool to derive highly consistent data from free text.
Large language models (LLMs) able to thoroughly interpret natural language are now available. These models have achieved remarkable performance on a wide range of language-related tasks and are capable of extracting relevant information even from conversational texts. There are, however, significant limitations in using these models for a project such as eCREAM. These models are trained on general knowledge, but in areas such as the medical domain they do not function as well. Furthermore, the largest and best-performing models are proprietary, and their integration with other software is therefore expensive and introduces privacy issues.
Primary objective:

To develop and validate a state-of-the-art language model for the six languages of the project (English, Greek, Italian, Polish, Slovakian, and Slovenian) that is able to interpret EHR contents and to extract crucial information from them.
See the protocol for more information:
Study protocol - NLP-DeVal: Development and validation of a natural language processing tool to enable clinical research in emergency and acute care medicine: retrospective cohort study.
Bertolini G, Ghilardi GI, Pandolfini C, Nattino G, Lavelli A, Moretti F (2024).